
April 08, 2025 Published by Enrico Palumbo, Gustavo Penha, Andreas Damianou, José Luis Redondo García, Timothy Christopher Heath, Alice Wang, Hugues Bouchard, Mounia Lalmas
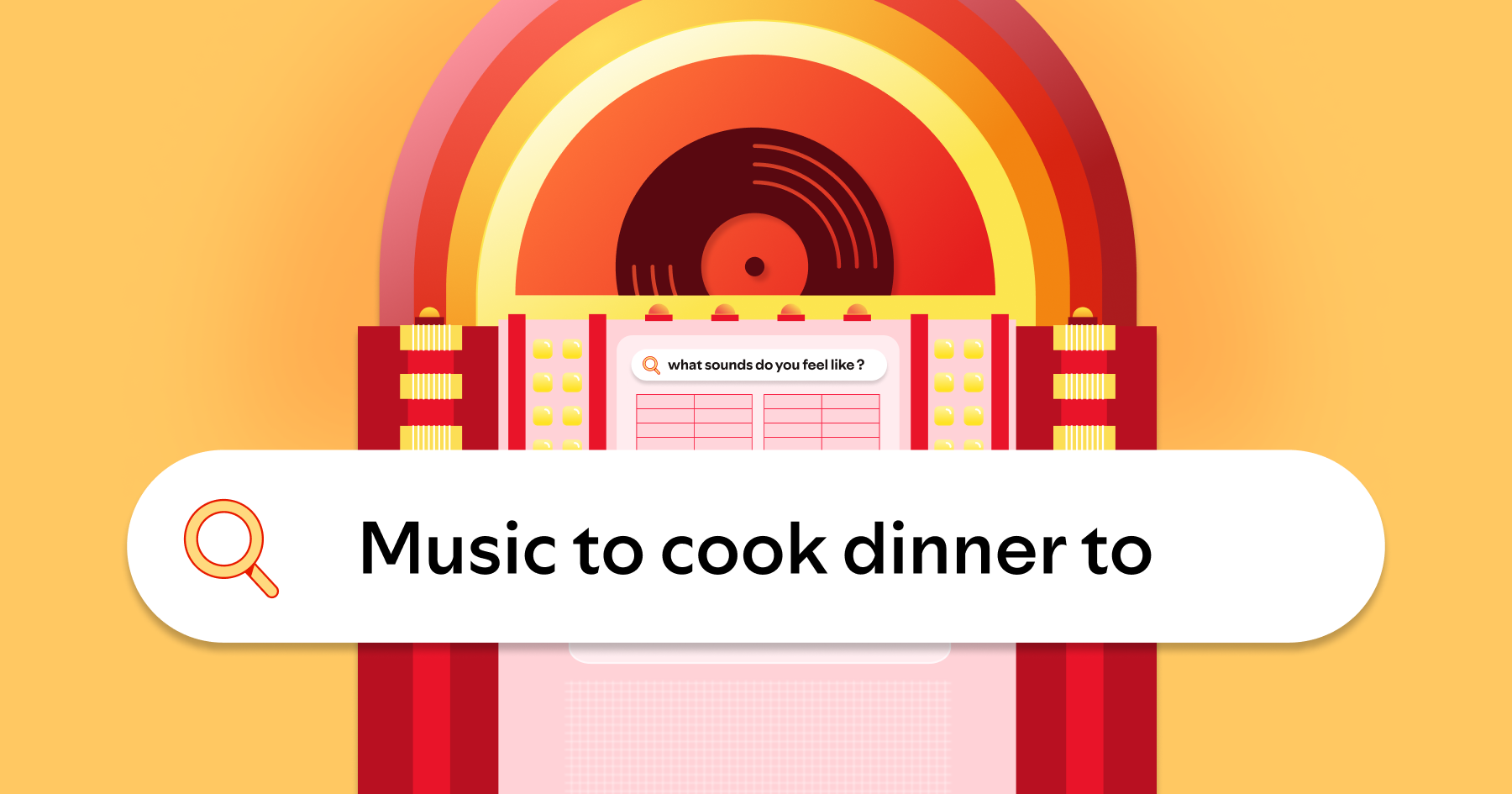
Imagine asking your music app to “play some old-school rock ballads to relax” and immediately receiving the right observe suggestion. This sort of customized music discovery is the objective of Text2Tracks, a brand new system primarily based on generative AI designed to enhance how music is really helpful primarily based in your selection of phrases.
Why Current Methods Fall Short
Some current music suggestion options—reminiscent of these seen in Spotify—have begun to include off-the-shelf Large Language Models (LLMs) to course of pure language prompts. For instance, LLMs can generate artist names and track titles as their output (e.g. User: “Can you recommend some chill songs for me? LLM: “Sure, I can recommend
While intuitive, the method of figuring out tracks by way of their title has a number of limitations:
- Song titles are usually not descriptors: Song titles don’t all the time replicate the temper or model of the music. For instance, two songs with related names may have fully completely different genres or vibes.
- Song titles may be ambiguous: Songs typically have a number of variations (e.g., stay, acoustic, remastered), and it’s not all the time clear which one to advocate.
- Slow and expensive: Song titles and artist names may be fairly lengthy. Since LLMs generate their responses one token (i.e. piece of phrase) at a time, producing the complete title is computationally costly and time-consuming.
To overcome these challenges, we developed Text2Tracks, a mannequin that introduces a extra environment friendly and efficient strategy to advocate music by fine-tuning an LLM to instantly generate optimized observe identifiers (IDs) given a music suggestion immediate.
Figure 1: Text2Tracks supplies music suggestion by producing observe IDs which can be related to the person’s immediate.
How Text2Tracks Works
Rather than producing precise track titles and matching them to a database, Text2Tracks makes use of Generative Retrieval: a Generative AI method the place the system is educated to generate observe IDs instantly from textual content prompts. These IDs instantly determine songs within the music catalog and permit for quicker, extra correct suggestions.
Here’s the way it works (Fig. 1):
- Experimenting with completely different methods to create observe identifiers, the playlist dataset is pre-processed acquiring pairs of playlist titles and observe IDs
- An LLM is fine-tuned on the pre-processed playlist information, enabling Text2Tracks to study the connection between person requests (like “chill acoustic vibes”) and the songs that match them.
- At suggestion time, the system generates a set of IDs for songs that match the question utilizing a diversified beam search technique (i.e., a decoding method that balances relevance with range by exploring a number of believable sequences, reasonably than simply the top-ranked ones). This avoids the necessity for a further search step, whereas nonetheless offering a related and diversified set of suggestions.
How Text2Tracks is Trained and Tested
A key distinction between Text2Tracks and off-the-shelf LLMs is that Text2Tracks undergoes an in depth fine-tuning course of tailor-made particularly for music suggestion. We fine-tune the mannequin utilizing a big corpus of chosen playlists, the place lengthy and descriptive playlist titles (e.g., “energetic rock vibes,” “chill relaxing at the beach”) function a proxy for pure language music prompts. This permits the mannequin to study associations between textual descriptions and related observe alternatives.
To consider the effectiveness of this method, we examine the mannequin’s suggestions to playlists curated by skilled editors, utilizing the hits@10 metric to measure how typically the mannequin’s prime predictions align with professional alternatives. This fine-tuning step helps the mannequin higher seize the nuances of music-related language and generate extra related and contextually acceptable observe suggestions.
The Role of Track IDs
One of probably the most progressive features of Text2Tracks is the way it assigns IDs to songs. We experimented with three completely different methods to seek out the easiest way to symbolize tracks (Tab. 1):
- Content-Based IDs: Use metadata like artist names and track titles to construct the ID (Artist Track Name). While easy and strong as a baseline, this technique faces the challenges talked about above within the “Why Current Methods Fall Short” part.
- Integer-Based IDs: Assign distinctive integers to songs, both in a naive approach the place every observe corresponds to a special integer (Track Integer) or in a structured approach making use of the artist-track hierarchy (Artist Track Integer). This method is simple to implement however could miss extra fine-grained relationships between related tracks, because it solely depends on the observe’s artist as metadata.
- Semantic (Learned) IDs: use vector discretization methods to create IDs primarily based on track traits, reminiscent of style, temper, model. For instance, two vacation songs may share a part of their ID (“<0><1>” and “<0><2>”), indicating their similarity. These identifiers may be seen as observe “zip codes”, figuring out parts of a vector area the place a observe lives. We examined two completely different vector areas, one primarily based on textual content embeddings (i.e. embedding titles of playlists the place the observe seems within the coaching set) and one primarily based on collaborative filtering embeddings (i.e. constructed mining patterns of songs showing collectively in playlists).
We discovered that Semantic IDs constructed on prime of collaborative filtering vectors labored the very best. This highlights the flexibility of Semantic IDs and of the underlying vector area to seize extra nuances in observe representations, leading to a better accuracy within the remaining set of suggestions. The Artist Track Integer technique got here in second, which is outstanding contemplating it’s comparatively easy to implement—it solely makes use of the artist and observe and doesn’t want an embedding area. This reveals how vital the artist-track hierarchy is for modeling observe identifiers and the way a lot it could possibly assist the mannequin study to make good observe suggestions.
It can also be price noting that the Artist Track Integer outperforms the Artist Track Name technique, which is usually utilized in many eventualities, reminiscent of off-the-shelf LLM suggestions. This additional underscores the benefit of constructing structured observe identifiers reasonably than figuring out songs by way of their titles.
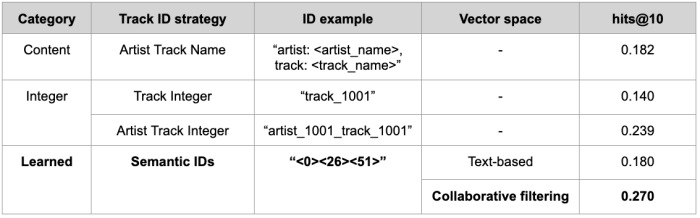
Tab. 1: hits@10 for various methods to create observe identifiers within the generative retrieval setting
How Text2Tracks Compare to Other Systems
We in contrast Text2Tracks to different textual suggestion strategies, together with:
- Bi-encoders: transformer fashions that detect semantic matches between textual content prompts and songs. In the zero-shot situation, we merely immediate the bi-encoder to match the question and the tracks. In the fine-tuned one, we fine-tune the bi-encoder on the identical playlist information we use for Text2Tracks.
- BM25: A state-of-the-art keyword-based retrieval system.
Tab. 2: hits@10 for Text2Tracks in comparison with well-known textual content retrieval approaches
We see that Text2Tracks considerably outperformed these baselines, reaching 127% higher accuracy than the closest competitor. When wanting into why it really works so nicely, we qualitatively observe that it tends to advocate extremely related and canonical tracks for broad prompts, matching the person’s expectation. For occasion, when requested for “Christmas classics”, Text2Tracks reliably retrieved probably the most iconic vacation tracks, whereas different programs supplied extra generic vacation songs.
Looking forward
In this submit, we launched Text2Tracks, a brand new method to music suggestion that generates observe IDs instantly from a person immediate, delivering quicker and extra correct outcomes. Trained on playlist information and leveraging Semantic IDs constructed on prime of collaborative filtering embeddings, Text2Tracks outperforms present programs for prompt-based music suggestion.
These outcomes spotlight the potential of generative fashions to complement music search and suggestion on Spotify. We are excited to proceed advancing these applied sciences, with the objective of constructing a unified generative AI system that enhances a number of aspects of music suggestion. Ultimately, we goal to make music discovery extra customized, intuitive, and pleasing for everybody.
For extra info, please discuss with our paper:
Text2Tracks: Prompt-based Music Recommendation by way of Generative Retrieval
Enrico Palumbo, Gustavo Penha, Andreas Damianou, José Luis Redondo García, Timothy Christopher Heath, Alice Wang, Hugues Bouchard, Mounia Lalmas.