
June 06, 2024 Published by Spotify Research
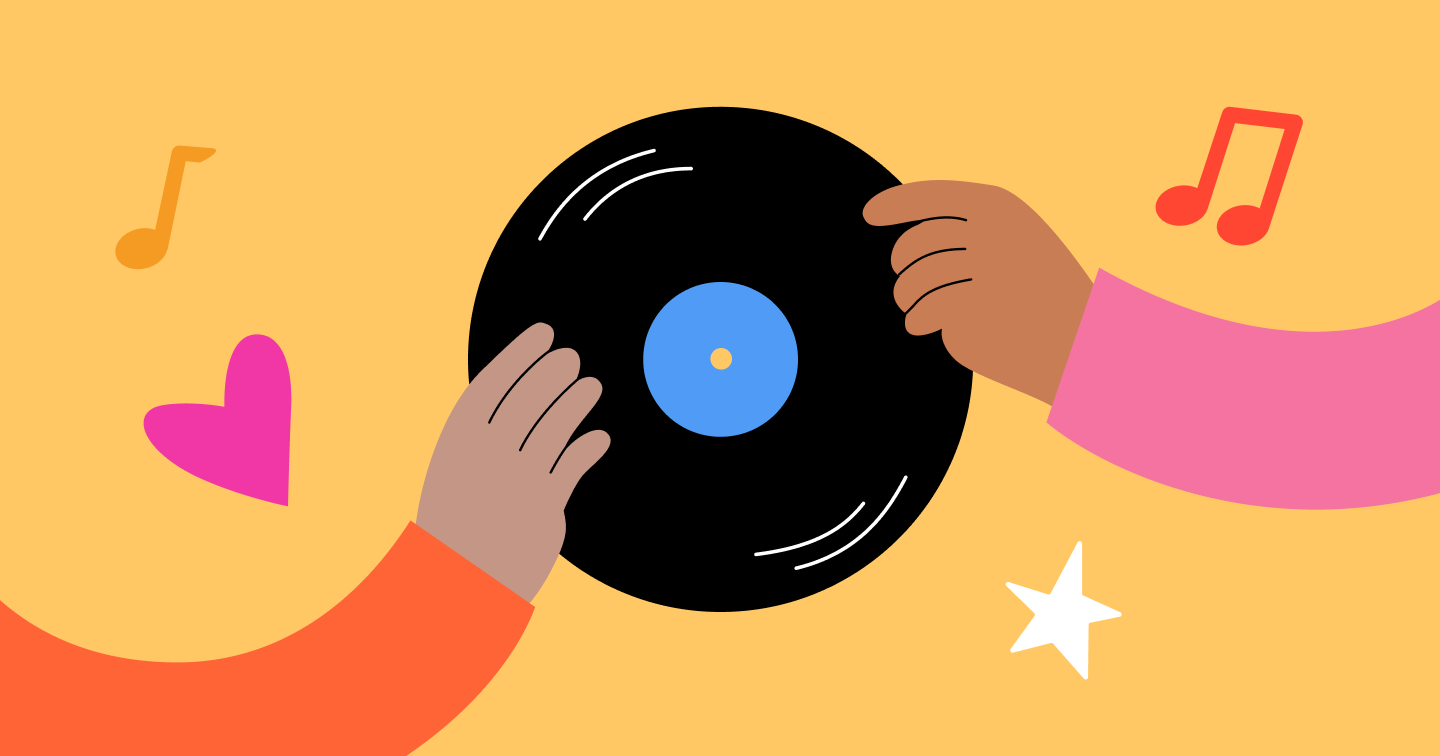
tldr – The extensive number of causes folks hearken to music embrace each particular person motivations, like self-awareness and temper regulation, in addition to social motivations, comparable to demonstrating belonging to a gaggle or feeling linked to associates. Music advice programs implicitly are inclined to concentrate on particular person motivations for music listening. In this work, we discover the opportunity of a recommender system geared in direction of a selected social motivation: the will to hearken to music that’s common inside one’s neighborhood. We body a advice activity round this want and suggest a easy methodology for satisfying it. Using an analysis metric devised for this activity, we study our method’s effectiveness throughout cultures.
Social motivations for music listening
Music is a social exercise that connects folks globally by live shows, karaoke, and shared listening experiences. Even when people hearken to music alone, their social motivations affect their selections, typically main them to hunt music that fosters a way of neighborhood. This want for shared experiences is clear when folks deliberately hearken to music that’s common inside their neighborhood, which differs from listening aimed toward temper regulation or self-awareness. Such communal listening lays the muse for social bonding by shared musical familiarity. Although these motivations are common, analysis reveals they’re particularly vital in collectivist cultures. Collectivism is a standard means of characterizing cultures that emphasize obligation to in-group and sustaining concord, in distinction to individualism, which prioritizes independence and uniqueness.
There has been little exploration of how suggestions ought to account for the social motivations behind music consumption. While algorithmic advice programs typically draw inspiration from social processes, they appear to disregard essential parts of social worth. Collaborative filtering, for instance, makes use of the similarity between folks’s musical style as the idea for suggestions. But this widespread association ceaselessly assumes that the particular person receiving the advice doesn’t care who these different individuals are and that it doesn’t matter when their listening passed off.
A advice system framed round music’s social features may require completely different assumptions. We discover such a system, specializing in the purpose of serving to folks join with their communities by shared musical familiarity. To accomplish this purpose, we try and determine and suggest newly launched songs which are “trending” inside a person’s neighborhood.
Recommending trending music inside a neighborhood
To suggest music that’s trending inside a neighborhood, we’d like two fundamental parts: a technique of figuring out what music is trending, and a mannequin of person communities. To examine this drawback, we use a dataset of the listening exercise of 100,000 Spotify customers from 20 international locations over a interval of two months.
First, to determine what music is trending, we have a look at how consciousness of a track spreads inside a neighborhood over time. We measure this by taking a look at what fraction of a neighborhood has listened to a track, daily, because the track’s launch. In the determine beneath, for an instance group of customers, we will see how consciousness evolves over time for a number of newly-released songs. Shorter traces replicate songs launched nearer to the top of our two month pattern interval. Some songs acquire recognition rapidly, whereas others take longer however in the end obtain better recognition. Many customers don’t present consciousness of common songs till over a month after their launch. Our advice system goals to assist these customers uncover these songs earlier.
We use the thought of the gradual unfold of track consciousness to implement a heuristic method to advice. On day x after the discharge of a brand new track, if the fraction of a gaggle of customers who’ve listened to the track exceeds threshold y, we determine the track as trending; we then suggest the track to all customers within the group who haven’t but listened to it.
Next, we mannequin the communities the place members care about maintaining with every others’ musical preferences. We infer these communities from customers’ listening habits and demographic attributes, utilizing info comparable to age, gender, nation of residence, and the languages and genres wherein customers hearken to music. We use combos of those attributes to section customers into distinct teams.
We conduct a collection of offline experiments to look at the effectiveness of this method and the way it responds to 3 variables: the attributes used to section customers; and the variety of days to attend and the fraction of customers required so as to make a advice. To consider these experiments, we concentrate on two essential qualities of socially-motivated music suggestions: relevance and social worth.
To measure relevance, we compute the precision of our suggestions in comparison with customers’ implicit suggestions on held-out information. In different phrases, if we suggest a trending track at day x to a person who would later have listened to the track on their very own, we take into account the advice a hit.
Listening to the identical music as one’s neighborhood implies that the listening should happen across the similar time. To seize the social worth of this exercise, we thus concentrate on the timeliness of our suggestions. The earlier a person learns concerning the music trending of their neighborhood, the extra social worth the advice gives. We measure timeliness by counting the variety of days between day x, once we suggest a track, and when a person would have found it on their very own based on our pattern information. This metric estimates the period of time our method “saved” the person from not understanding a couple of trending track.
By contemplating each the timeliness and precision of suggestions, we will assess how effectively our method meets two key wants related to socially-motivated music listening: figuring out the music that’s related to each a person and their neighborhood, and figuring out it promptly sufficient to offer social worth.
Findings
How effectively does the proposed music advice system fulfill customers’ pursuits and supply social worth? We take into account the outcomes of our offline experimentation from a number of views.
First, we discover a clear tradeoff between two parameters of our system: the variety of days to attend and the fraction of customers required so as to make a advice. Waiting longer to evaluate a track’s trendiness results in suggestions which are extra exact however much less well timed. Similarly, the fraction of customers we require to make a advice could be understood as a measure of strictness; greater values for this threshold result in fewer, extra correct suggestions.
Next, we evaluate completely different attributes by way of how effectively they approximate real-world musical communities. The best suggestions emerge from segmenting customers by their style preferences, nation of residence, and age. These outcomes correspond to the instinct that geography, tradition, and style all play a task in defining folks’s musical communities. It additionally echoes findings in earlier analysis that folks’s relationship to new music modifications with age.
Our method to advice was notably simpler in international locations characterised by a better diploma of collectivism. This consequence suggests the actual significance of socially-motivated listening in collectivist cultures, and it’s per earlier analysis on collectivism and listening patterns. These findings additionally level to the broader significance of cultural variations to music advice programs.
The analysis metric of timeliness affords a helpful lens for inspecting the social worth of a music advice, emphasizing that when a advice happens could be as essential as what is beneficial. Studying precisely how the timeliness of suggestions interprets into worth for listeners presents a chance for additional analysis.
For extra info, please see our paper:
Socially-Motivated Music Recommendation
Ben Lacker, Samuel Way