
September 5, 2024
On Spotify’s Analytics Platform, we’re devoted to constructing merchandise that empower knowledge practitioners to find, analyze, and share insights — suppose notebooks, dashboards, and metrics. To create options that join with our customers, it’s essential for us to first perceive who our customers are. However, the evolving panorama of information roles presents a problem.
In the previous decade, we’ve noticed an trade development towards consolidating numerous roles beneath the Data Scientist umbrella. Yet not all knowledge scientists have the identical wants, and plenty of workers working with knowledge don’t even maintain that title. While we adopted the time period “data practitioner” as a workaround, it proved too broad to successfully information our product growth. So we constructed a framework to raised perceive who our knowledge practitioners are. Enter personas.
To create personas, we took a mixed-methods strategy, which means we blended qualitative insights from in-depth interviews with quantitative evaluation of worker knowledge, permitting us to establish clusters of shared behaviors. We pushed for personas that had been each interpretable and relevant in order that product leaders might simply grasp them and use them to make tactical selections.
This work resulted in six distinct personas that compose the info practitioner universe at Spotify, together with a number of learnings related to all insights practitioners.
Curious to see the personas? Skip to the tip!
- Don’t simply facilitate collaboration between Data Science and Research by decreasing obstacles. Instead, erase the obstacles between knowledge science and person analysis.
While mixed-methods analysis generally entails one technique following one other, we discovered success in having an iterative loop — utilizing the qualitative work to tell the quantitative work, then the quantitative work to tell the qualitative work, and so forth, till we’re glad with the tip outcome. After a collection of person interviews, we created our first model of the quantitative guidelines that might inform persona classification. We then continued to interview extra customers to assist us validate our classifications, add depth to our information of the archetypes, and break ties to enhance the foundations we had been growing. This turned an iterative means of qualitative and quantitative validation, the place every person interview we carried out resulted in both validation or tweaks to the proposed quantitative guidelines.
- Don’t simply share findings and ship the deck round. Create momentum by brazenly discussing tailor-made suggestions together with your stakeholders and connecting them to product initiatives.
A standard problem to foundational work may be activating the work and actioning on the findings. Stakeholders could also be questioning: What will we do now that we all know this?
To encourage motion, we created devoted slides in our share-outs highlighting the personas for every workforce to concentrate on. These suggestions had been open for dialogue however gave place to begin to have interaction with the content material. We additionally supplied options on how the personas might inform future product technique.
With these slides as primers, we witnessed our groups study a typical language and understanding of who we had been constructing for. Teammates used the brand new vocabulary to speak about our customers throughout sprints and planning. Over the primary quarter, the framework went from being a dialog starter to changing into the muse for a way we set objectives and metrics.
- Don’t simply write the analysis plan and go off to work. Engage together with your stakeholders from begin to end.
For something however the smallest questions, knowledge scientists and person researchers tend to withdraw into the analysis course of and emerge eventually with ultimate solutions, indifferent from altering necessities throughout the analysis interval. Keeping our stakeholders concerned all through the undertaking diminished this threat.
Before kicking off the undertaking, we aligned on the background and scope of the work and remained open to stakeholder suggestions. To simply observe alongside throughout the undertaking, we created a devoted Slack channel for the analysis, to share updates and anecdotes on the fly. We additionally opened person interviews for commentary throughout the whole product space, no matter squad and function.
We additionally discovered that foundational work with longer timelines benefitted from sharing early learnings at key milestones. Midway by means of the undertaking, we shared “Postcards from the Field” — uncooked, unsynthesized qualitative knowledge that stored stakeholders up to the mark with the analysis. We additionally shared previews of the personas with our product space leads at later milestones to collect suggestions.
- Don’t take a fancy quantitative strategy for its personal sake. Keep your strategy easy and clear.
We used a rule-based strategy to categorise Spotifiers into personas. (For instance: “Employee has used dashboarding tools more than 90% of data scientists.”) Keeping the strategy easy made our outcomes simply interpretable, clear, and reliable to our stakeholders.
- Don’t simply transfer on to a different undertaking. Instead, discover alternatives to increase the work past the unique scope of the undertaking.
To make sure the personas could be used amongst our groups going ahead, we constructed a dataset to categorise each inside person at Spotify right into a persona every day. This dataset has not solely turn into useful for recruitment for future initiatives, but additionally provides extra coloration to our product space metrics. We at the moment are in a position to phase all our key outcomes by these persona varieties and discover person behaviors for every group.
Because this work is massive in scale and constructed foundational information throughout our product space, we needed to be sure that the result was straightforward to confer with and share. To make the personas extra memorable, we collaborated with our design companions to create Spotify-approved illustrations, including a face to every one. Building robust visible artifacts inspired higher longevity and shareability.
If you’re employed within the discipline, chances are you’ll be asking your self: So which knowledge persona am I?
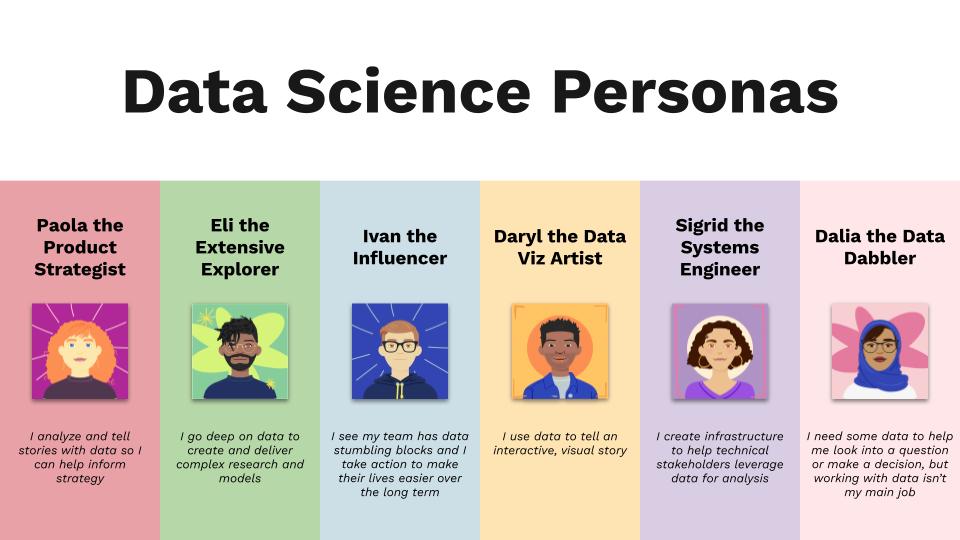
Paola the Product Strategist: Paolas are the commonest knowledge science persona at Spotify. They analyze and inform tales with knowledge to assist inform technique. They sometimes companion carefully with non-technical stakeholders. Outside Spotify they maintain titles like Product Analyst or Data Scientist, Analytics.
Eli the Extensive Explorer: Elis are the second-most-common persona sort at Spotify. These are knowledge scientists, machine studying engineers, or analysis scientists who go deep on knowledge to ship complicated analysis and fashions. This persona brings collectively the standard knowledge science function with MLE and related roles.
Ivan the Influencer: Ivans are leaders who help their knowledge groups and have affect over finest practices and processes. They could also be workforce leads or managers.
Daryl the Data Viz Artist: Daryls use knowledge to inform interactive, visible tales and spend most of their time creating dashboards to share with non-technical stakeholders. This function is a distinct segment at many firms and should not even have its personal title.
Sigrid the Systems Engineer: Sigrids are engineers who create infrastructure to assist technical stakeholders leverage knowledge for evaluation. Data Engineer or Analytics Engineer are frequent titles.
Dalia the Data Dabbler: Dalias aren’t knowledge scientists by title however work with knowledge to carry out evaluation or make selections. They sometimes discover simple knowledge questions, usually with technical help from the opposite personas.
–
Did one of many personas resonate with you? We discovered that the majority Spotify knowledge practitioners associated carefully to 1 persona, however some workers reported becoming a hybrid of two personas.
Tags: Data, engineering tradition