
November 24, 2022 Published by Ciarán M. Gilligan-Lee, Mounia Lalmas and Olivier Jeunen
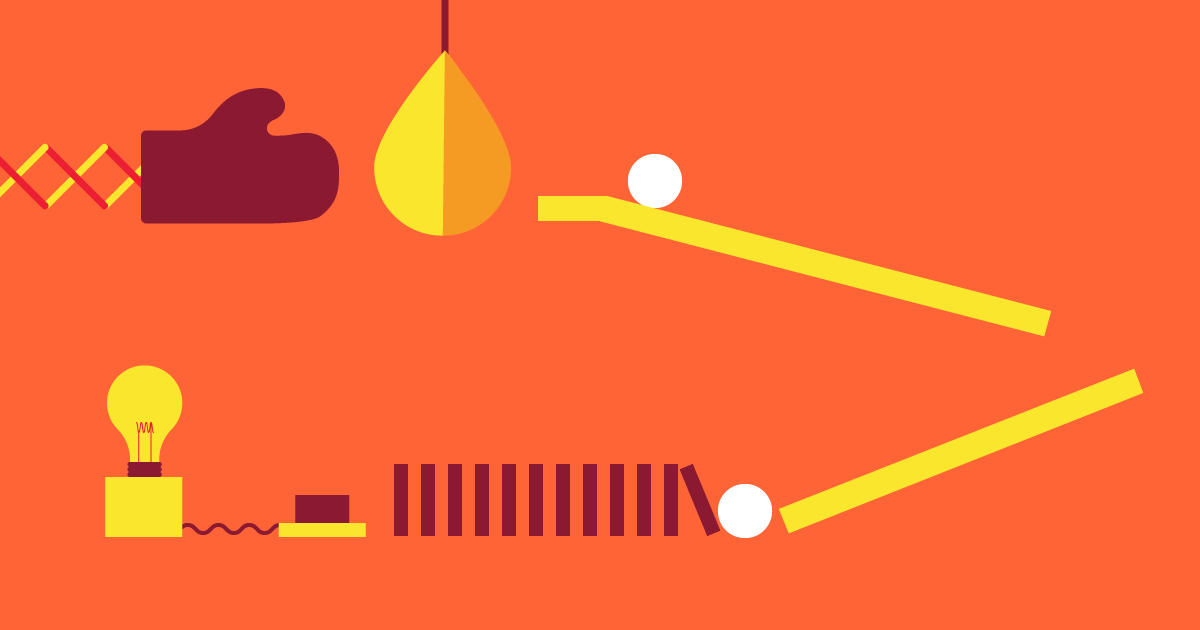
What can we do after we can’t do an A/B check?
There are many A/B assessments we would prefer to run, however that are too technically difficult, dangerous when it comes to person affect and even not possible to carry out. For occasion, within the traditional instance of whether or not smoking causes lung most cancers, forcing a randomly chosen group of individuals to smoke is unethical if we imagine it would harm their well being. In the context of expertise firms, if we need to perceive if app crashes trigger customers to churn, we must randomly choose a subgroup of customers and crash their apps on objective – not one thing we might need to think about given we don’t need to break their belief.
With no prospect of testing and a transparent threat of deceptive outcomes from observational information, what are we to do? Good solutions to those questions may inform decision-making in some ways, serving to us perceive what sort of content material we must always promote to realize the very best enterprise outcomes, or how we must always prioritise app adjustments and fixes, and so forth. Fortunately the causal inference toolkit can present actionable solutions about trigger and impact from observational information like these (and assumptions that should be rigorously scrutinised for every utility).
In the purposes we have now mentioned so far, solely a single intervention is feasible at a given time, or interventions are utilized one after one other in a sequential method. However, in some vital areas, a number of interventions are concurrently utilized. For occasion, in drugs, sufferers that possess many commodities could must be concurrently handled with a number of prescriptions; in computational promoting, folks could also be focused by a number of concurrent campaigns; and in dietetics, the dietary content material of meals may be thought-about a joint intervention from which we want to study the consequences of particular person dietary parts. Moreover, in the course of the pandemic, many interventions have been utilized on the identical time, equivalent to masks carrying, working from dwelling, closure of faculties, and so forth.
How can we study the affect of particular person interventions from such joint interventional information? This is the query we addressed in our paper.
Disentangling the impacts of a number of, interacting therapies
Disentangling the consequences of single interventions from collectively utilized interventions is a difficult process–particularly as concurrently utilized interventions can work together, resulting in penalties not seen when contemplating single interventions individually. This drawback is made more durable nonetheless by the attainable presence of unobserved confounders, which affect each therapies and final result. Our paper addresses this problem, by aiming to study the impact of a single-intervention from each observational information and collectively utilized units of interventions.
Formalising the issue
Identifiability is a basic idea in parametric statistics that pertains to the portions that may, or can’t, be discovered from information. An estimand is claimed to be identifiable from particular varieties of information whether it is theoretically attainable to study this estimand, given infinite samples from the info.
If two causal fashions coincide on stated information then they have to coincide on the worth of the estimand in query. Hence if one finds two causal fashions which agree on stated information, however disagree on the estimand, then the estimand just isn’t identifiable until additional restrictions are imposed.
Formally, the query we deal with is that this: Given samples from the info regimes that induce

when can we study the conditional common results of single interventions, proven under?

We show that this isn’t typically attainable. That is, with out restrictions on the causal mannequin, single-intervention results can’t be recognized from observations and joint-interventions.
However, we do present identification proofs demonstrating it may be achieved in sure courses of non-linear steady causal fashions with additive multivariate Gaussian noise–even within the presence of unobserved confounders. This fairly weak additive noise assumption is prevalent within the causal inference and discovery literature. Importantly, we present how you can incorporate noticed covariates, which may be high-dimensional, and therefore study heterogeneous remedy results for single-interventions.
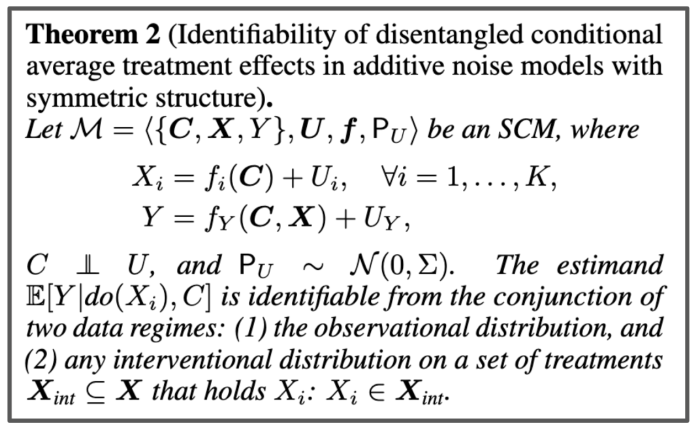
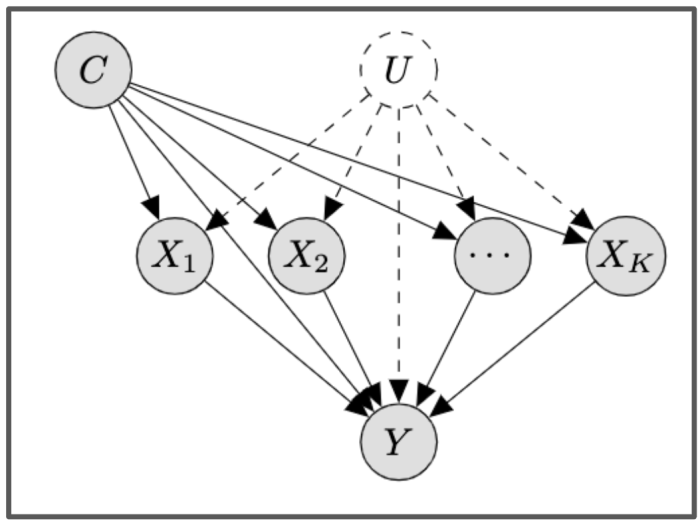
Estimation algorithm and empirical validation
In addition to proving theoretically that causal disentanglement is feasible given our assumptions, our paper additionally gives an algorithm to study the parameters of the proposed causal mannequin and disentangle single interventions from joint interventions. Experimenting on artificial information, we confirmed this algorithm can precisely estimate unseen units of interventions.
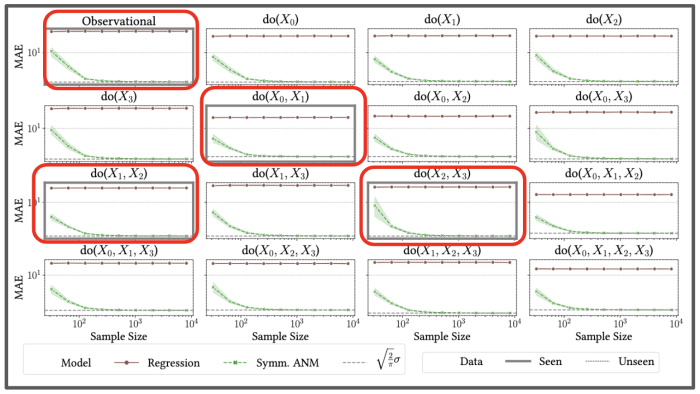
We additionally confirmed that our methodology is constant beneath various ranges of unobserved confounding by testing it on semi-synthetic setup on real-world information from the International Stroke Trial database. This was a big, randomised trial of as much as 14 days of antithrombotic remedy after stroke onset. There are two attainable therapies: the aspirin allocation dosage, and the heparin allocation dosage. The purpose is to grasp the consequences of those therapies on a composite final result, a steady worth in [0,1] predicting the chance of sufferers’ restoration.
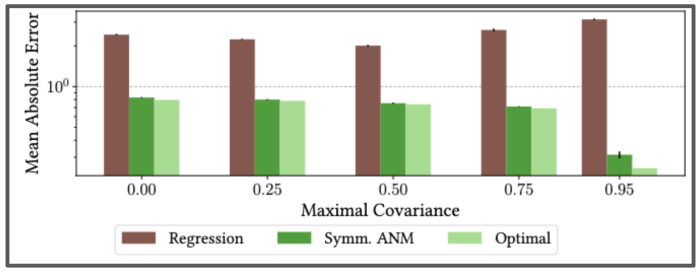
Conclusion
Our work was motivated by the necessity for strategies that may disentangle the consequences of single interventions from collectively utilized interventions. As a number of interventions can work together in probably complicated methods, it is a difficult process; much more so within the presence of unobserved confounders. The first results of our paper was a proof that such disentanglement just isn’t attainable within the normal setting, even after we prohibit the affect of the unobserved confounders to be additive in nature. Our foremost end result nevertheless, was to supply a proof of identifiability within the affordable additive noise mannequin setting. Additionally, we confirmed how you can incorporate noticed covariates, and have empirically demonstrated our methodology. For extra details about paper:
Disentangling Causal Effects from Sets of Interventions within the Presence of Unobserved Confounders
Olivier Jeunen, Ciarán M. Gilligan-Lee, Rishabh Mehrotra and Mounia Lalmas
NeurIPS 2022