
September 29, 2022
TL;DR Understanding algorithmic affect is crucial to constructing a platform that serves a whole lot of tens of millions of listeners and creators on daily basis. Our strategy features a mixture of centralized and distributed efforts, which drives adoption of finest practices throughout all the group — from researchers and information scientists to the engineer pushing the code.
How we strategy algorithmic accountability
At Spotify, our aim is to create outcomes that encourage advice variety and supply new alternatives for creators and customers to find and join. This additionally signifies that we’re taking accountability for the affect that we’ve on creators, listeners, and communities as a platform, and ensures we will assess and be accountable for his or her affect. To attain this aim, we consider and mitigate in opposition to potential algorithmic inequities and harms, and attempt for extra transparency about our affect. Our strategy is to watch Spotify as an entire, in addition to allow product groups, who know their merchandise finest, to optimize for algorithmic accountability.
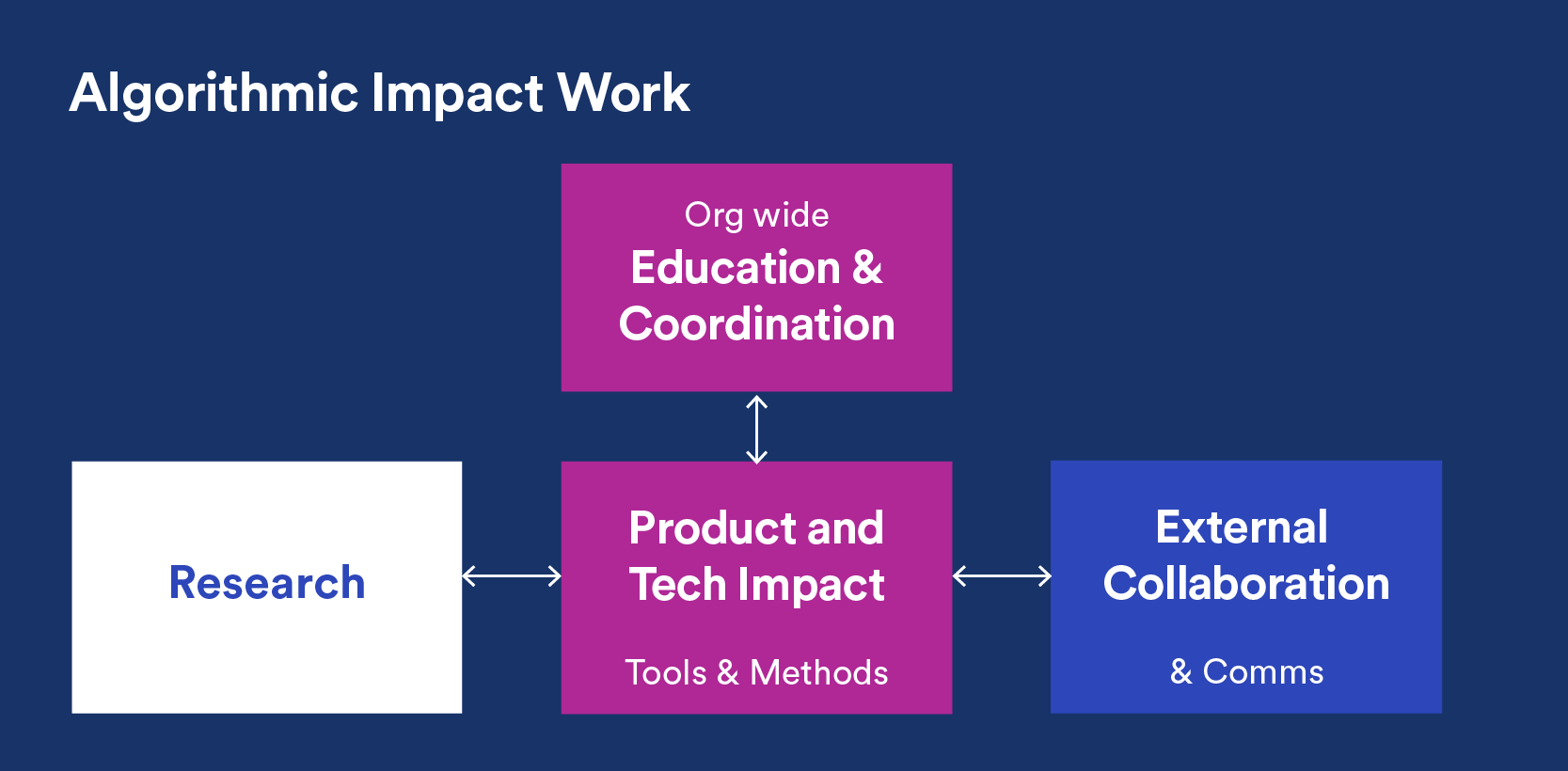
Broadly, Spotify’s strategy to algorithmic accountability covers three areas:
- Analysis: How we mix our analysis strategies throughout disciplines with case research to make sure high-quality information choices and equitable algorithmic outcomes.
- Product and tech affect: How we work with our product groups to find out which modifications can really deal with algorithmic affect in apply. This requires creating consciousness of challenges and alternatives to advance accountability in our merchandise via schooling and coordination.
- Exterior collaboration: How we share and collaborate with different researchers and practitioners locally at massive.
Analysis
From privacy-aware deep dives into metrics and strategies to product-focused case research, our algorithmic accountability analysis, which we’ve been doing for a number of years, serves as the inspiration for understanding the problems that we’re most involved with at Spotify.
Some examples of our revealed analysis embrace: investigating illustration of feminine artists in streaming (2020), assessing the accessibility of voice interfaces (2018), exploring how underserved podcasts can attain their potential viewers (2021), and reflecting on the challenges in assessing algorithmic bias itself (2018).
Product and tech affect
Studying from analysis needs to be translated into instruments and strategies that work in apply. This requires direct collaboration with product groups.
For instance, the algorithmic affect analysis neighborhood has begun providing ML equity toolkits, which intention to show the newest equity analysis into APIs that anybody can use. When these first appeared, our researchers have been excited concerning the potential of this new class of instrument and labored along with our product groups to judge which of them might work with Spotify’s advice techniques. Because of this collaboration between analysis and product, we discovered {that a} continued effort is required for these analysis toolkits to be efficient from a sensible standpoint together with the place they wanted probably the most enchancment.
Advancing algorithmic accountability requires area expertise and experience to be helpful in the actual world. We obtain this via organization-wide schooling and coordination. Researchers in our algorithmic accountability effort collaborate not solely with our different product and analysis groups, but in addition with our editorial groups and different area specialists, to ensure we perceive the medium-specific challenges inherent to music, podcasting, and different domains. Cross-functional collaboration with our authorized and privateness groups ensures that affect assessments are in step with our strict privateness requirements and relevant legal guidelines.
Exterior collaboration
An important piece of the puzzle is studying from different researchers and practitioners. We contribute to the broader neighborhood by publishing our algorithmic accountability analysis, sharing classes and finest practices, and facilitating collaborations with exterior companions. Making our work public is a crucial means of fostering knowledgeable dialog and pushing the trade ahead.
How we expanded our efforts
Leveraging the strategy above, we’ve (and proceed to) evolve and develop our work to create a platform that’s safer, extra truthful, and extra inclusive for listeners and creators:
- We constructed out the capability of our crew to centrally develop strategies and observe our affect. This core crew of qualitative and quantitative researchers, information scientists, and pure language processing specialists assists product and coverage groups throughout the corporate to evaluate and deal with unintended dangerous outcomes, together with stopping the exacerbation of current inequities via suggestions.
- We helped create an ecosystem throughout the corporate for advancing accountable suggestions and enhancing algorithmic techniques. This contains launching focused work with particular product groups, in addition to collaborations with machine studying, search, and product groups that assist to construct user-facing techniques.
- We launched governance, centralized steering and finest practices to facilitate safer approaches to personalization, information utilization, and content material advice and discovery. This additionally creates house to additional examine inequitable outcomes for creators and communities by offering pointers and finest practices to mitigate algorithmic harms.
How we use algorithmic affect assessments at Spotify
Educational and trade researchers advocate auditing algorithmic techniques for potential harms to allow safer experiences. Based mostly on that groundwork, we’ve established a course of for algorithmic affect assessments (AIA) for each music and podcasts. These inner audits are a necessary studying instrument for our groups at Spotify. However AIAs are only one a part of placing algorithmic accountability into apply. AIAs are like maps, giving us an summary of a system and highlighting hotspots the place product changes or analysis into directional modifications are wanted.
Proudly owning the product and the method
Our AIAs immediate product house owners to assessment their techniques and create product roadmaps with the intention to take into account potential points that will affect listeners and creators. The assessments will not be meant to function formal code audits for every system. As a substitute, the assessments name consideration to the place deeper investigation and work could also be wanted, akin to product-specific steering, deeper technical evaluation, or exterior assessment. Identical to merchandise, affect assessments, auditing strategies and requirements additionally want iteration following a primary part rollout. By way of additional refinement and piloting, we count on to proceed to learn the way the affect evaluation course of and translation between cross-functional groups might be improved.
Rising accountability and visibility
For product house owners, performing an evaluation not solely reinforces finest practices resulting in actionable subsequent steps, it additionally will increase accountability since audits are shared with stakeholders throughout the group and work is captured and prioritized on roadmaps. Taken collectively, AIAs assist us see the place we’ve probably the most work to do as an organization, together with added visibility into groups’ product plans. Together with serving to groups define and prioritize their work, assessments additionally assist set up roles and duties. The AIA course of contains the appointment of formal product companions to work with the core algorithmic accountability crew.
Outcomes to this point
In lower than a yr, we’ve educated a big variety of Spotifiers and assessed greater than 100 techniques throughout the corporate that play a job in our personalization and advice work. The Spotify expertise is produced by the interactions of many techniques, from fashions that advocate your favourite style of music to particular playlists. Because of these efforts, we’ve:
- Established formal work streams that led to enhancements in suggestions and search
- Improved information availability to raised observe platform affect
- Decreased information utilization to what’s really vital for higher product outcomes for listeners and/or creators
- Helped groups implement further security mechanisms to keep away from unintended amplification
- Knowledgeable efforts to deal with harmful, misleading, and delicate content material, as described in our Platform Guidelines
Now that we’ve added AIAs as one other instrument for our groups to make use of, what has the method taught us about operationalizing algorithmic accountability in apply?
5 classes from implementing algorithmic affect assessments
AIAs will proceed to assist us perceive the place there may be new work to be accomplished and permit us to enhance our strategies and instruments. And whereas direct solutions to product questions will not be at all times out there — neither within the analysis literature nor in commercially out there tooling — we wish to share 5 classes that emerged from the method of implementing assessments.
1. Turning rules into apply requires continuous funding
Operationalizing rules and ideas into day-to-day product improvement and engineering practices requires sensible constructions, clear steering, and playbooks for particular challenges encountered in apply. This work won’t ever be “accomplished.” As we proceed to construct new merchandise, replace our current merchandise, and make developments in machine studying and algorithmic techniques, our want to judge our techniques at scale will proceed to develop. In some circumstances, we are going to want new instruments and strategies past what’s at present out there in both the analysis or trade communities round accountable machine studying. For others, we might want to discover new methods to automate the work we do to scale back the burden on product liaisons.
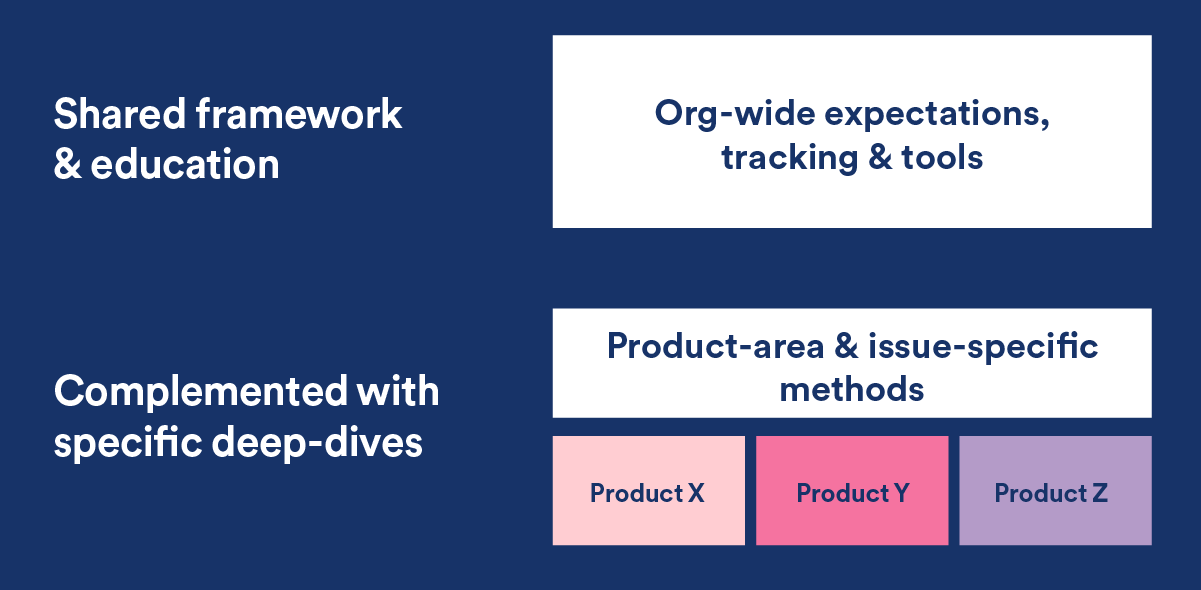
2. Algorithmic accountability can’t simply be the job of 1 crew
It’s crucial that algorithmic accountability efforts will not be owned by only one crew — enchancment requires each technical and organizational change. Duty efforts require that each crew perceive their roles and priorities. We’ve targeted on organizing folks and processes in addition to offering organizational assist, together with creating house for greater than machine studying. This effort has enabled us to extend transparency and assist make algorithmic accountability ‘enterprise as regular’ for a whole lot of Spotifiers throughout the corporate.
3. There isn’t any singular ‘Spotify algorithm’, so we want a holistic view
Product experiences depend upon the interdependence of many techniques, algorithms, and behaviors working collectively. Which means assessing and altering one system could not have the meant affect. Coordination throughout groups, enterprise capabilities, and ranges of the group is important to create a extra holistic view of the place there is perhaps crucial dependencies and strategies to trace throughout several types of techniques.
4. Analysis requires inner and exterior views
Inner auditing processes like AIAs are complemented by exterior auditing, together with work with educational, trade, and civil society teams. Our crew collaborates with and learns from specialists in domains past simply design, engineering, and product. Our work with Spotify’s Variety, Inclusion & Belonging and editorial groups are essential, as is our partnership with members of the Spotify Security Advisory Council. We additionally work with researchers from disciplines akin to social work, human-computer interplay, info science, pc science, privateness, regulation, and coverage. Translation between disciplines might be difficult and takes time, however is essential and definitely worth the funding.
5. Downside fixing requires translation throughout sectors and organizational constructions
Timelines between product improvement and insights from the sphere might be very totally different, which signifies that it’s essential to set expectations that not all algorithmic affect issues might be simply ‘solved,’ particularly not by technical means. Evaluation efforts ought to be knowledgeable by analysis on what issues in a particular area (e.g. illustration in music or podcasting, in addition to the affect of audio tradition on society), but in addition inner analysis on tips on how to finest create construction and assist inside your current organizations and improvement processes. That’s the reason we’re actively investing in information, analysis, and infrastructure to have the ability to observe our affect higher, and sharing finest practices with others throughout academia and trade. Our crew additionally interprets insights and studying via the broader subject, together with participation in educational and trade conferences and workshops, in addition to via formal collaboration with impartial researchers.
The place we’re going
That is an ever-evolving subject, and as members of this trade, it’s our accountability to work collaboratively to proceed to determine and deal with these subjects. Simply as content material itself and the trade that surrounds it continues to evolve, our work in algorithmic affect will probably be iterative, knowledgeable, and targeted on enabling the very best outcomes for listeners and creators.
To study extra about our analysis and product work, go to: analysis.atspotify.com/algorithmic-responsibility.
We’re grateful to members of the Spotify Security Advisory Council for his or her considerate enter and ongoing suggestions associated to our Algorithmic Affect Evaluation efforts.
Tags: engineering management