
July 19, 2022 Published by Shahrzad Nazeri, Sravana Reddy, Joana Correia, Jussi Karlgren, Rosie Jones
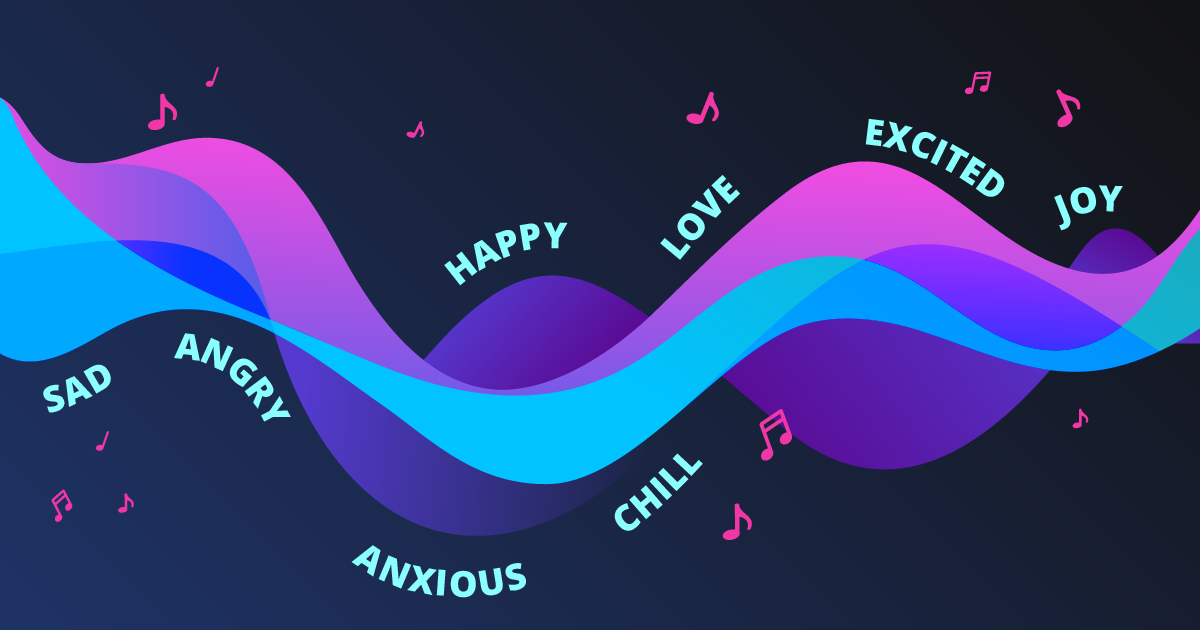
Song lyrics make an vital contribution to the musical expertise, offering us with wealthy tales and messages that artists need to convey by way of their music. They affect the perceived temper of a track, alongside the acoustic contents (together with the rhythm, concord, and melody) of the track. In some circumstances, these two parts – lyrics and acoustics – work collectively to determine a cohesive temper; and in others, every part gives its personal contribution to the general temper of the track.
Let’s think about an instance of a track, the place its lyrics speak in regards to the finish of a relationship, and counsel moods associated to unhappiness, longing and heartbreak, whereas on the similar time, its acoustics have a well-known chord development, and considerably excessive tempo, suggesting calm and upbeat moods. This situation is just not an exception, the truth is, a current evaluation of the lyrics and acoustics of common music identifies a development the place track lyrics have been getting sadder within the final three a long time, whereas on the similar time, the songs additionally change into extra “danceable” and “relaxed”.
In our current ICWSM paper, we got down to examine the affiliation between track lyrics and temper descriptors, i.e. the phrases that describe affectual qualities of a track. To this finish, we conduct a knowledge pushed evaluation utilizing state-of-the-art machine studying (ML), and pure language processing (NLP) methods, to check how lyrics contribute to the understanding of temper, as outlined collaboratively by the playlisting conduct of Spotify customers.
This work is motivated by our need to enhance the Spotify expertise, particularly in relation to music search, discovery and proposals. From the search and discovery perspective, we need to allow search based mostly on temper descriptors within the Spotify app, for instance by permitting customers to seek for “happy songs”. Additionally, from the suggestions facet, we wish to have the ability to advocate new songs to customers that present comparable units of moods customers would possibly already like.
At the identical time, this work is pushed by the analysis query, “How much do the lyrics and acoustics of a song each contribute to understanding of the song’s mood?”.
Data
In this work we used a set of slightly below 1 million songs.
The temper descriptors for this set of songs included phrases like “chill”, “sad”, “happy”, “love”, and “exciting”. They will not be restricted to a particular part-of-speech, protecting adjectives (“sad”, “somber”, and many others), nouns (“motivation”, “love”, and many others.) and verbs (“reminisce”, “fantasize”, and many others.).
The affiliation between a track and a temper descriptor was calculated utilizing collaborative knowledge, by “wisdom of the crowd”. More particularly, these relationships had been derived from Spotify playlists’ titles and descriptions, by measuring the co-occurrence of a given track in a playlist, and the goal temper descriptor in its title or description.
Experiments and outcomes
We tackled a lot of experiments geared toward finding out the contribution of lyrics and acoustics to the temper of a track. In this weblog put up we summarize a few of the most related ones we carried out within the scope of this downside, and for extra particulars, we invite you to learn the complete paper linked on the finish of this put up.
To perceive the contribution of lyrics, acoustics, or mixture of lyrics and acoustics to the temper of a track, we used a number of ML classifiers to foretell temper descriptors, every skilled on options extracted from completely different modalities: acoustic, lyrics, and hybrid. Then, we carried out an evaluation of the completely different fashions, and in contrast their outcomes.
Lyrics and temper descriptors: We begin by finding out the connection between track lyrics and temper descriptors. To this finish, we prepare a number of fashions that leverage track lyrics alone, and never audio. These fashions could be broadly categorized into two distinct studying paradigms: zero-shot studying, and fine-tuned fashions. For the previous, we benefit from fashions skilled on both pure language inference (NLI), or subsequent sentence prediction (NSP) duties, to symbolize the lyrics and predict their relationship to temper descriptors. For the latter, we use both a bag-of-words (BoW) mannequin, or options extracted from transformer-based fashions to symbolize the lyrics, earlier than modeling their relationship to temper descriptors.
Acoustics and temper descriptors: To deal with modeling the connection between the acoustics solely of songs and moods, we prepare one mannequin on options extracted from the Spotify API, which captures acoustic data that describes audio, and songs particularly, when it comes to a number of acoustic traits similar to beat power, danceability, vitality, and others. These options had been then modeled to foretell the affiliation of a track’s audio to a temper.
Lyrics, acoustics and temper descriptors: Finally, we discover two hybrid approaches, which seize data from each the lyrics and the acoustics of songs to foretell their affiliation with any given temper. One represents the track (lyrics, and acoustics) by concatenating the bag of phrases illustration of the lyrics and the Spotify API acoustic options (‘Hybrid-BoW’).
The different creates a hybrid illustration of a track based mostly on the options obtained by a superb tuned transformer mannequin, and the hidden illustration of the identical acoustic options because the Hybrid-BoW mannequin, obtained by feeding them right into a multilayer perceptron (MLP) (‘Hybrid-NLI’). This hybrid illustration is handed right into a classification head to generate predictions. The picture beneath reveals a diagram with the structure of the Hybrid-NLI mannequin.
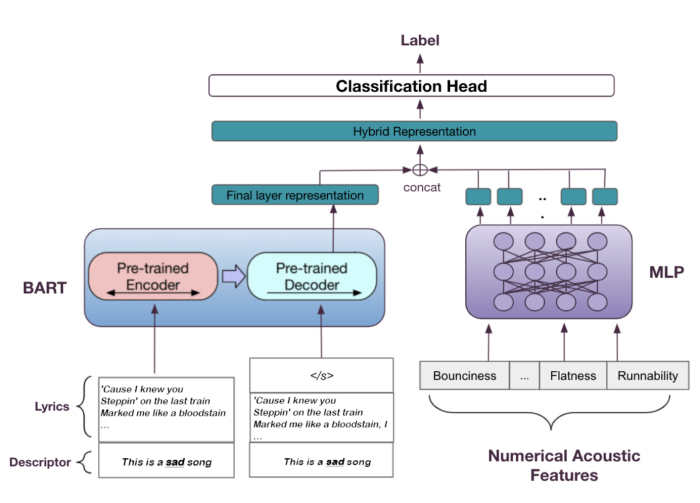
After coaching all of the fashions – based mostly on acoustic, lyrics or hybrid options – we take a look at them on our Spotify dataset. The efficiency of all of the fashions is reported in precision, recall and F1-score, within the desk beneath.
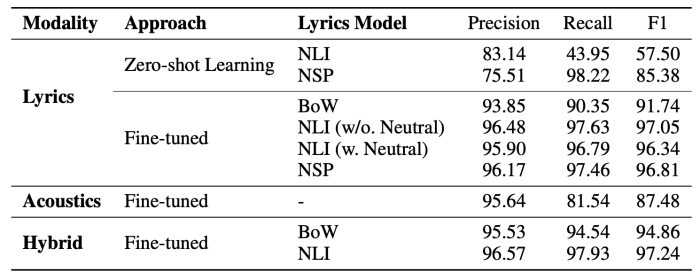
Conclusions
With these experiments, we noticed, based mostly on one of the best performing mannequin for every modality, that lyrics play a much bigger position than acoustics to determine the temper of a track. At the identical time, by trying on the efficiency of the hybrid fashions, significantly the Hybrid-NLI, we noticed that by combining data extracted from the 2 modalities – lyrics and acoustics – we will finest predict the connection between a track and a given temper. This outcome strengthened our preliminary speculation that lyrics and acoustics work collectively, both in concord or by complementing one another to determine the temper of a track.
Overall, the gathering of outcomes obtained from these experiments are encouraging, in that they present us that it’s doable to study patterns that correlate songs to temper descriptors – a extremely private and subjective job.
We additional broke down the issue of discovering patterns between songs and moods, and in contrast the efficiency of our fashions at predicting completely different moods, observing that some are rather more ambiguous than others. We additionally in contrast the efficiency of our mannequin to that of human annotators. These and different experiments could be present in our paper linked on the finish of this put up.
Summary
In this work we’ve got seemed on the affiliation between track lyrics and temper descriptors by way of a data-driven evaluation of Spotify playlists. We took benefit of state-of-the-art pure language processing fashions based mostly on transformers to study the affiliation between track lyrics and temper descriptors, based mostly on the co-occurrence of temper descriptors and songs in Spotify playlists. We’ve additionally decoupled the contribution of track acoustics and lyrics to determine a track’s temper, and noticed that the relative significance of lyrics for temper prediction as compared with acoustics will depend on the particular temper. These, and some extra experiments, could be present in our paper right here:
The Contribution of Lyrics and Acoustics to Collaborative Understanding of Mood
Shahrzad Naseri, Sravana Reddy, Joana Correia, Jussi Karlgren, Rosie Jones
ICWSM 2022